Top Ways Predictive Analytics Is Used in Insurance Operations
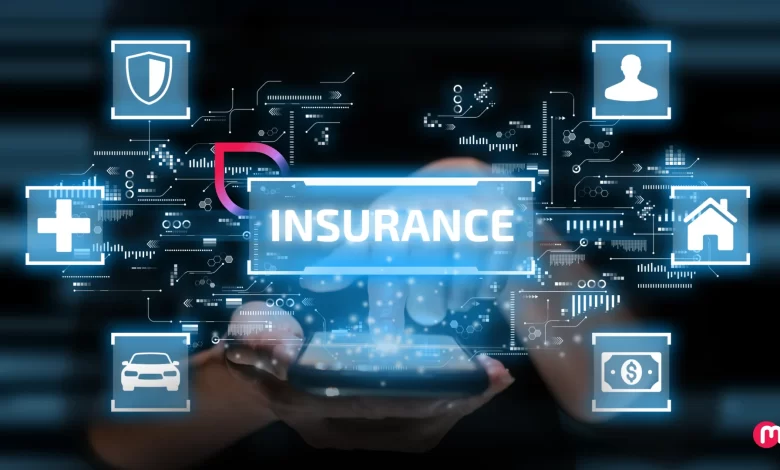
Predictive analytics in insurance is revolutionizing the way insurers operate by transforming vast data into actionable insights to forecast risks, enhance customer experiences, and more. This advanced technology has been in use for decades, evolving from manual calculations to sophisticated predictive models powered by machine learning and real-time data insights.
A recent survey by Willis Towers Watson revealed that 67% of insurers have seen a reduction in policy issuance and underwriting expenses after adopting advanced predictive analytics. Additionally, 60% of insurers reported an increase in sales and profitability, while 83% believe that predictive analytics plays a crucial role in underwriting processes.
But how exactly does predictive analytics work in insurance, and what value does it offer? Let’s delve into the key aspects of predictive analytics in insurance, including its implementation steps, challenges, and solutions.
Predictive analytics in insurance leverages massive data and statistical modeling to forecast future events and outcomes. By understanding customer behavior and anticipating potential risks, insurers can make informed decisions to improve business operations and profitability. This technology plays a critical role in various insurance sectors, including health, life, and auto insurance, as well as property and casualty insurance.
To implement predictive analytics successfully, insurers must undergo a digital transformation strategy that supports data integration and utilizes AI models to generate human-centric insights. Data engineering services can assist in preparing data and setting up the architecture for predictive analytics.
The process of predictive analytics in insurance involves data ingestion from diverse sources, data processing, feature engineering, pattern recognition, model application, scoring, segmentation, decision-making, and continuous model improvement. This closed-loop system ensures that historical and real-time data flow through analytical models to deliver accurate predictions.
The benefits of predictive analytics in insurance are significant, including improved risk management, faster claims processing, early fraud detection, customer retention uplift, personalized customer experiences, dynamic pricing strategies, enhanced operational efficiency, and a competitive advantage in the market.
Key use cases of predictive analytics in insurance range from identifying high-risk drivers for auto insurance to predicting health conditions in life and health insurance, reducing fraud, forecasting natural disaster impacts, predicting customer lifetime value, and enhancing cross-sell and upsell strategies.
Top applications of predictive analytics in insurance include hyper-accurate risk assessment and mitigation, personalized customer experiences, real-time fraud detection, pricing and premium optimization, and customer churn prediction and retention strategies.
Implementing predictive analytics in insurance operations requires a strategic approach, including defining business objectives, collecting and integrating data, choosing the right tools and technologies, building and training predictive models, ensuring regulatory compliance, deploying and integrating models, and monitoring and refining them continuously.
Challenges in introducing predictive analytics in insurance operations include data silos, talent gaps, and regulatory hurdles. Solutions include implementing centralized data warehouses, upskilling the existing team, and aligning model design with industry-specific regulations.
Change management strategies with the implementation of predictive models involve securing leadership buy-in, providing employee training and upskilling, starting with a phased rollout, and maintaining transparent communication with stakeholders.
Real-world examples of predictive analytics in insurance include Progressive’s Snapshot Program for auto insurance and John Hancock’s use of predictive analytics in life insurance to optimize retirement savings plans.
Future trends in predictive analytics for insurance include achieving hyper-personalization, leveraging ethical AI, embracing embedded insurance, implementing AI-driven underwriting, and using generative AI for predictive scenario modeling.
Partnering with a data science services company like MindInventory can help insurance businesses successfully implement predictive analytics, leverage industry-specific expertise, ensure compliance with regulations, and future-proof analytics with cloud engineering services.
In conclusion, predictive analytics is reshaping the insurance industry by enabling insurers to make data-driven decisions, optimize processes, and enhance customer experiences. By embracing this advanced technology, insurance companies can stay ahead of the curve and thrive in an increasingly competitive market.